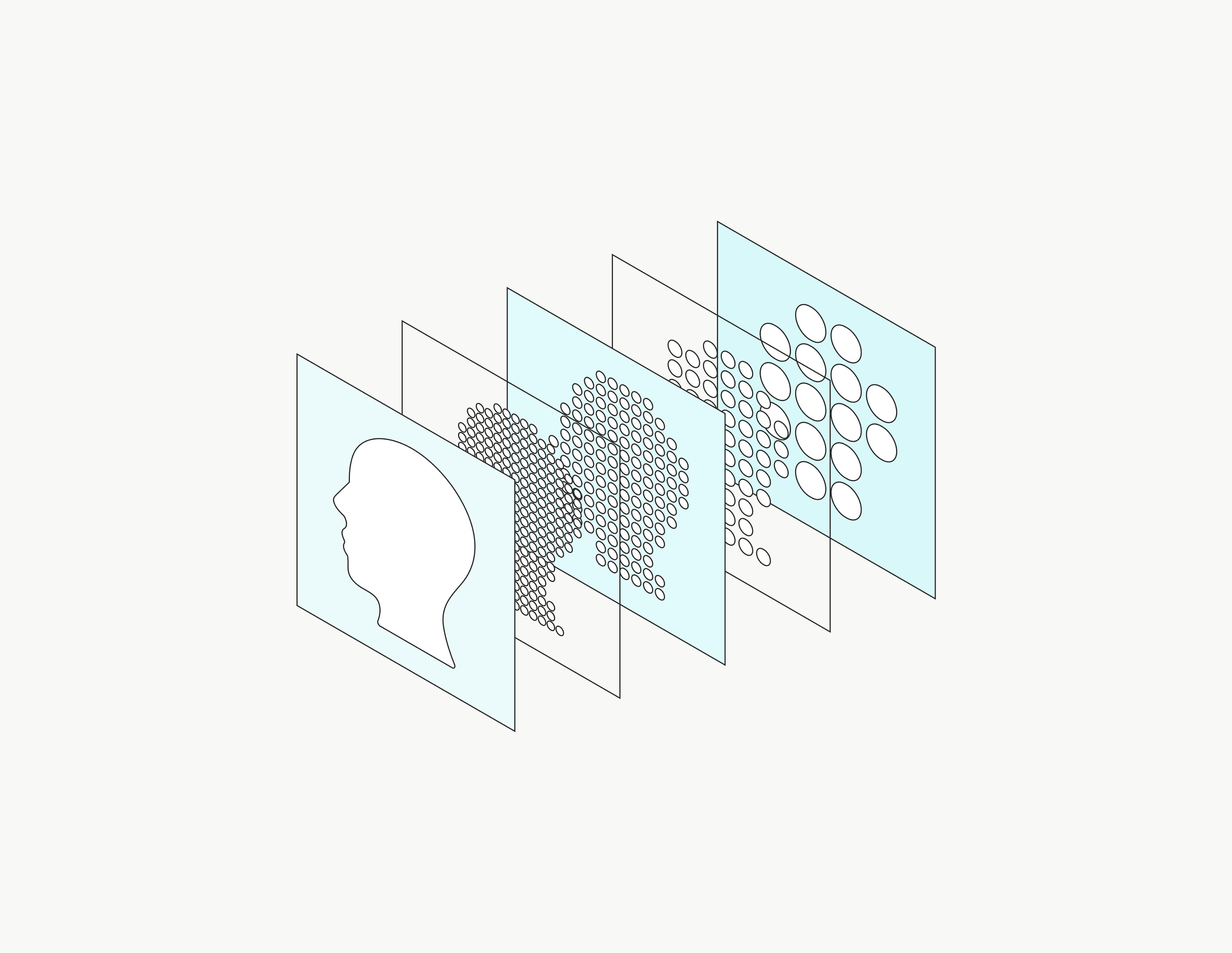
As AI advances, data anonymization plays an increasingly important role in safeguarding privacy.
That’s because large amounts of data are needed to train AI. Today, that data identifies people, their patterns and behaviors, their likes and dislikes, etc., and more must be done to protect humans as a result of these AI-driven privacy realities.
Anonymizing data, whether it’s for AI training or creating the tools that keep humans safe in the age of AI, is essential. But what is anonymization and how does it work to protect individual privacy?
How World anonymizes data
Data anonymization means changing data so that it cannot be effectively linked back to an individual.
There are different ways to anonymize data. World achieves anonymization through the use of advanced cryptography known as Multi-Party Computation, which we build upon to create Anonymized Multi-Party Computation (AMPC). Here’s how it works.
- When a human verifies their World ID at an Orb, photos are taken of their face and eyes. A numerical code (iris code) is abstracted from the photo of their eyes. This code can be used to prove they are a unique human and images used to create the code are only stored on the individual’s device, never on the Orb.
- The iris code is then cryptographically converted into multiple encrypted fragments that each reveal nothing about the individual or the iris code and cannot be effectively linked back to the individual.
- These fragments are stored in databases operated by trusted third parties such as Nethermind and the Friedrich Alexander Universität Erlangen-Nürnberg in Germany.
As a result of this cryptographic process, the anonymized data cannot effectively be linked back to an individual. This means a person using a verified World ID can prove they’re uniquely human online while maintaining their anonymity.
Establishing anonymization standards
What it means for data to be anonymized is a question that continues to divide technologists, engineers, policy makers and regulators, and today there is still no consensus on what it takes to achieve data anonymization. While not a new issue, many existing laws do not address this key definitional question.
Some people believe that it must be effectively impossible to link the data back to a person, while others take the approach that it must be completely, technically impossible to link the data back to a person without regard to the resources and the practical or technical difficulties in doing so.
This latter approach is problematic, since a standard based on technical impossibility even in hypothetical situations leads to nonsensical outcomes. It would mean that, even if the means to link the data back to a person are beyond the resources of any one entity, anonymization would still be impossible.
In a world where anonymizing data will help protect humans living and interacting with AI on a daily basis, having a clear and practically feasible definition of the term is critical. That’s why the World Foundation and other World Network contributors invested in creating a ground breaking, open source AMPC setup and are committed to working with technologists, engineers, policy makers and regulators to ensure that data anonymization is available as a key tool in the future.
Learn more
Download World’s privacy by design whitepaper here, and read World Foundation's response to BayLDA on the important issue of anonymization here.
To find out more about how World Foundation and TFH are working together with regulators to push for a more robust understanding and definition of anonymization, visit the World website or join the daily conversations on Twitter/X, WhatsApp, Discord, YouTube, Telegram and LinkedIn, or sign up for the blog newsletter at the bottom of this page.
Additional important information concerning privacy and anonymization is available in the World protocol whitepaper.
Disclaimer
The above content speaks only as of the date indicated. Further, it is subject to risks, uncertainties and assumptions, and so may be incorrect and may change without notice. A full disclaimer can be found in our Terms of Use and Important User Information can be found on our Risks page.